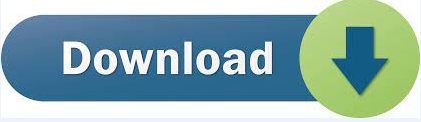

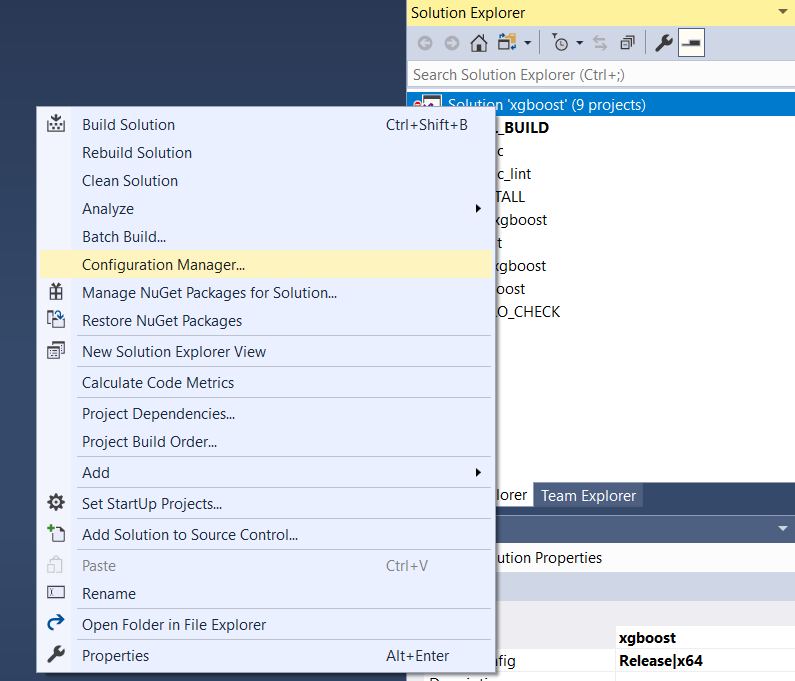
Congratulations on your new purchase! Also, I’m very sorry for all the trouble. R-release (arm64): xgboost_1.7.3.1.tgz, r-oldrel (arm64): xgboost_1.7.3.1.tgz, r-release (x86_64): xgboost_1.7.3.1.tgz, r-oldrel (x86_64): xgboost_1.7.3.1.tgzĪdapt4pv, alookr, audrex, autoBagging, autostats, bambu, BayesSpace, CausalGPS, causalweight, ccmap, CRE, creditmodel, dblr, EIX, fastrmodels, GeneralisedCovarianceMeasure, GNET2, GPCERF, infinityFlow, inTrees, irboost, latentFactoR, MBMethPred, mikropml, mixgb, modeltime, MSclassifR, nfl4th, nflfastR, nsga3, oncrawlR, personalized, predhy, predhy.GUI, predictoR, PriceIndices, promor, radiant.model, rminer, scDblFinder, scds, SELF, sentiment.ai, SHAPforxgboost, shapviz, simPop, surveyvoi, tidybins, traineR, trena, TSCI, tsensembler, twang, visaOTR, wactor, weightedGCM, xgb2sql, xrfīAGofT, bigsnpr, Boruta, breakDown, bundle, butcher, cfbfastR, ClassifyR, coefplot, cuda.ml, DALEXtra, DriveML, easyalluvial, embed, familiar, fastshap, fdm2id, FeatureHashing, FLAME, flashlight, forecastML, GenericML, lime, MachineShop, mcboost, metabolomicsR, miesmuschel, mistat, mistyR, mlflow, mlr, mlr3benchmark, mlr3hyperband, mlr3learners, mlr3tuning, mlr3tuningspaces, mlr3viz, modelplotr, modelStudio, modeltime.You bought/built a new PC and due to your work or personal preference, you use Windows 10. HighPerformanceComputing, MachineLearning, ModelDeployment, Survivalĭiscover your data XGBoost presentation XGBoost from JSON xgboost: eXtreme Gradient Boosting XGBoost contributors (base XGBoost implementation) The package is made to be extensible, so that users are also allowed to define Various objective functions, including regression, classification and ranking.
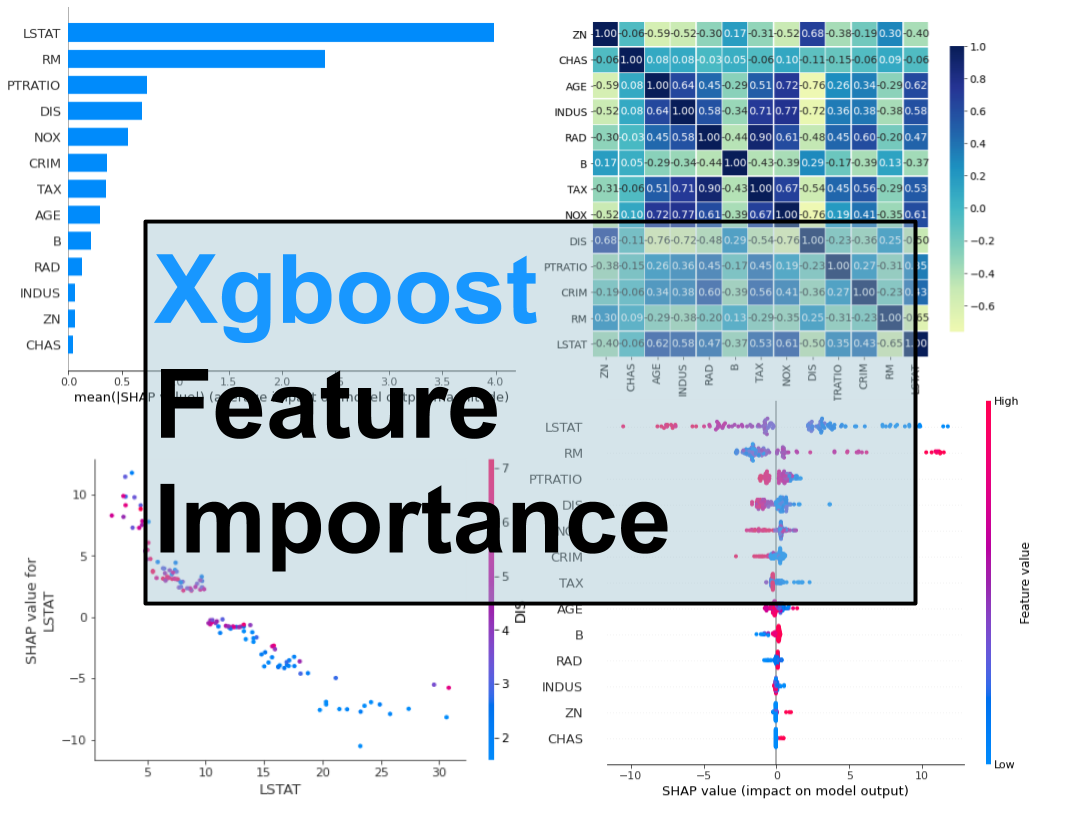
Times faster than existing gradient boosting packages. The package can automaticallyĭo parallel computation on a single machine which could be more than 10 Model solver and tree learning algorithms. Of the gradient boosting framework from Chen & Guestrin (2016). Extreme Gradient Boosting, which is an efficient implementation
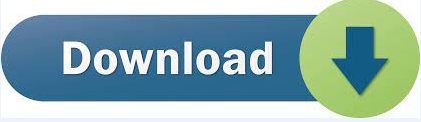